Making Meaningful Impact: Using Data Science for Social Good
By Jennifer Monahan
Imagine living in one half of a duplex. Though you maintain your part of the home, the other half of the building is abandoned and has fallen into disrepair. The roof is leaking. Unidentified critters have made a nest in the wall. Mold is creeping into the attic. Regardless of how well you keep up your personal living space, your home’s safety and value will be affected.
Abandoned buildings in disrepair pose a safety hazard and can have adverse effects on the structural integrity of adjacent residences – especially among the row homes that comprise the majority of housing units in Baltimore City, Maryland. Neighbors deal with rat infestations, have difficulty getting insurance, and experience damage to their own homes because of being attached to structures with severe roof damage.
These challenges are occurring at a city-wide scale in Baltimore, where the city’s Department of Housing & Community Development is tasked with assessing 15,000 vacant homes to identify and remediate roof damage. The problem is complex, systemic, and formidable.
Enter the Data Science for Social Good (DSSG) Summer Fellowship at Carnegie Mellon University (CMU).
Baltimore’s Department of Housing & Community Development partnered with DSSG to improve community safety and economic well-being by remediating buildings with roof damage in Baltimore. Aspiring data scientists from the DSSG team identified hazardous buildings with roof damage, then prioritized the most urgent needs for preventative interventions. Team member Chae Won Lee said one significant challenge was determining from the ground level whether a roof had damage. A second was the scope of work, with so many vacant homes in Baltimore to assess.
Abandoned buildings in disrepair pose a safety hazard and can have adverse effects on the structural integrity of adjacent residences – especially among the row homes that comprise the majority of housing units in Baltimore City, Maryland. Neighbors deal with rat infestations, have difficulty getting insurance, and experience damage to their own homes because of being attached to structures with severe roof damage.
These challenges are occurring at a city-wide scale in Baltimore, where the city’s Department of Housing & Community Development is tasked with assessing 15,000 vacant homes to identify and remediate roof damage. The problem is complex, systemic, and formidable.
Enter the Data Science for Social Good (DSSG) Summer Fellowship at Carnegie Mellon University (CMU).
Baltimore’s Department of Housing & Community Development partnered with DSSG to improve community safety and economic well-being by remediating buildings with roof damage in Baltimore. Aspiring data scientists from the DSSG team identified hazardous buildings with roof damage, then prioritized the most urgent needs for preventative interventions. Team member Chae Won Lee said one significant challenge was determining from the ground level whether a roof had damage. A second was the scope of work, with so many vacant homes in Baltimore to assess.
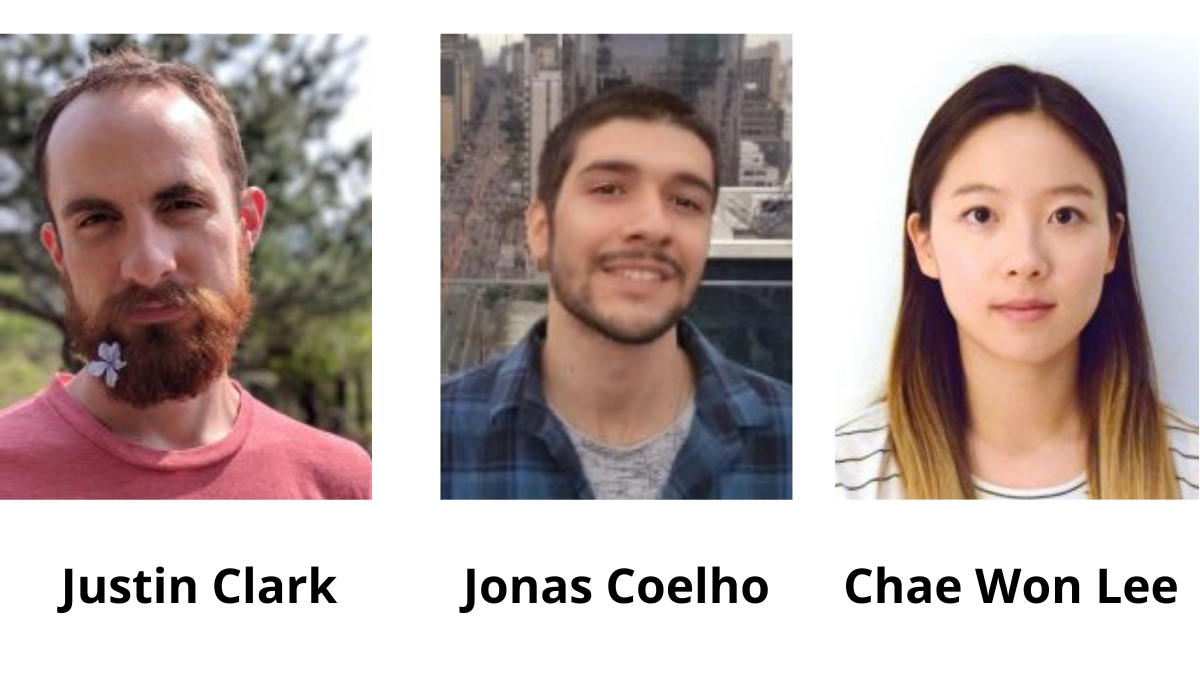
DSSG Fellows
Team members Justin Clark, Jonas Coelho de Barros, and Chae Won Lee created a successful system that used machine learning to assign a roof damage score to each address.
Lee and her project teammates, Justin Clark and Jonas Coelho de Barros, created a successful system that used machine learning to assign a roof damage score to each address. Incorporating data that included aerial images of the entire city, manual visual assessments of historical aerial inspections, housing inspection notes, details from 311 citizen’s hotline calls, and other information provided by the city, the team developed an AI system that effectively identified and prioritized structures with the most significant roof damage.
The prioritized list allows city inspectors to be more efficient and more equitable by focusing efforts on buildings with actual damage across neighborhoods and communities that are most impacted by this problem. The list can be regenerated each year with minimal manual effort. The system is more effective than relying on human observation in accurately identifying roof damage. Finally, the model eliminates potential bias by identifying roof damage equitably across neighborhoods. Ultimately, their solution has the potential to improve the lives of people in 5,000 households on city blocks with damaged roofs.
The Department of Housing & Community Development (DHCD) recently garnered an innovation award for the project’s impact.
The Baltimore roof initiative is just one example of the impact DSSG and CMU are having on communities locally, nationally, and internationally. In another project, DSSG Fellows worked to improve call routing for 988, the National Suicide Prevention Lifeline.
Picture someone you love suffering with depression or other mental health issues. They decide to reach out for help, and call the National Suicide Prevention Lifeline. They wait and wait for a person to answer the call, but after a few minutes, they hang up the phone.
An estimated 50 million people in the United States live with mental illness. The National Suicide Prevention Lifeline receives two million calls each year, which are routed to about 200 call centers around the country.
Team members Tejumade Afonja, Charles Cui, Paula Subías-Beltrán, and Irene Tang worked with Vibrant Emotional Health to address lengthy call wait times that result in nearly 20 percent of calls being abandoned before the callers receive help.
The prioritized list allows city inspectors to be more efficient and more equitable by focusing efforts on buildings with actual damage across neighborhoods and communities that are most impacted by this problem. The list can be regenerated each year with minimal manual effort. The system is more effective than relying on human observation in accurately identifying roof damage. Finally, the model eliminates potential bias by identifying roof damage equitably across neighborhoods. Ultimately, their solution has the potential to improve the lives of people in 5,000 households on city blocks with damaged roofs.
The Department of Housing & Community Development (DHCD) recently garnered an innovation award for the project’s impact.
The Baltimore roof initiative is just one example of the impact DSSG and CMU are having on communities locally, nationally, and internationally. In another project, DSSG Fellows worked to improve call routing for 988, the National Suicide Prevention Lifeline.
Picture someone you love suffering with depression or other mental health issues. They decide to reach out for help, and call the National Suicide Prevention Lifeline. They wait and wait for a person to answer the call, but after a few minutes, they hang up the phone.
An estimated 50 million people in the United States live with mental illness. The National Suicide Prevention Lifeline receives two million calls each year, which are routed to about 200 call centers around the country.
Team members Tejumade Afonja, Charles Cui, Paula Subías-Beltrán, and Irene Tang worked with Vibrant Emotional Health to address lengthy call wait times that result in nearly 20 percent of calls being abandoned before the callers receive help.
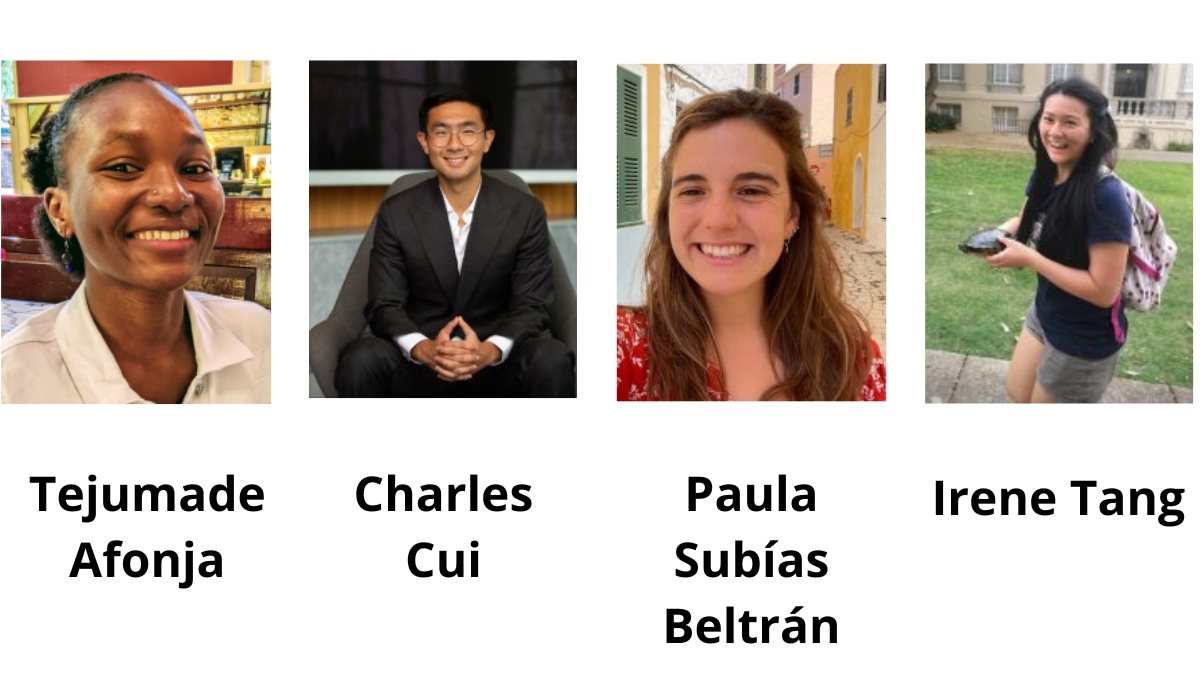
DSSG Fellows
Tejumade Afonja, Charles Cui, Paula Subías-Beltrán, and Irene Tang worked with Vibrant Emotional Health to address lengthy wait times for the National Suicide Prevention Lifeline.
Subías-Beltrán said that ideally, the team would need to know the current capacity of each call center, the current waiting time for each call center, and the length of time a caller is willing to wait – but none of that data was available to the network because of its distributed nature.
The team worked with the data available in the system to determine an alternative routing approach based on where each call came from, the call center where the calls were routed, the wait times, and whether the call was answered. They were able to create a model that predicted the likelihood that a call would be picked up at a specific call center at a given time. The team’s model has the potential to be better than the approach the organization had been using, and allowed the team to build a new routing simulator that can increase the connection rate for callers. That improvement means thousands of additional callers seeking mental health assistance may get the support they need in time. The change will ultimately save lives.
“The intersection of what I cared about and what I was good at – that’s the work I really wanted to do,” Ghani said. As chief data scientist for the Obama 2012 campaign, Ghani had had a taste of what it felt like to do work that made an impact on society.
He had an “a-ha moment” in 2013 during a talk to a group of CMU graduate students in machine learning (ML).
“I was trying to tell them about the intersection of ML and social issues,” Ghani said. “What I expected was that they knew about the social problems but didn’t find them interesting. What I heard that was a little bit surprising was that they didn’t realize there was this intersection, and that we could do something about those problems with these skills.”
At the same time, Ghani wondered why data and evidence were not used more often in government to solve societal problems. In talking with colleagues at government agencies and non-profits who worked on social issues, Ghani consistently heard one of three explanations.
The team worked with the data available in the system to determine an alternative routing approach based on where each call came from, the call center where the calls were routed, the wait times, and whether the call was answered. They were able to create a model that predicted the likelihood that a call would be picked up at a specific call center at a given time. The team’s model has the potential to be better than the approach the organization had been using, and allowed the team to build a new routing simulator that can increase the connection rate for callers. That improvement means thousands of additional callers seeking mental health assistance may get the support they need in time. The change will ultimately save lives.
How DSSG Came to Be
Rayid Ghani, Distinguished Career Professor in the Machine Learning Department and the Heinz College of Information Systems and Public Policy at CMU, created DSSG because he was looking to bridge a gap – for himself and for his students.“The intersection of what I cared about and what I was good at – that’s the work I really wanted to do,” Ghani said. As chief data scientist for the Obama 2012 campaign, Ghani had had a taste of what it felt like to do work that made an impact on society.
He had an “a-ha moment” in 2013 during a talk to a group of CMU graduate students in machine learning (ML).
“I was trying to tell them about the intersection of ML and social issues,” Ghani said. “What I expected was that they knew about the social problems but didn’t find them interesting. What I heard that was a little bit surprising was that they didn’t realize there was this intersection, and that we could do something about those problems with these skills.”
At the same time, Ghani wondered why data and evidence were not used more often in government to solve societal problems. In talking with colleagues at government agencies and non-profits who worked on social issues, Ghani consistently heard one of three explanations.
None of these complex problems can be solved by any discipline alone.Rayid Ghani
Some individuals were familiar with the concepts of ML and artificial intelligence (AI), but were not sure exactly how they could be used to address specific issues. Another group understood the capabilities of AI, but lacked staff skilled in using it. Finally, some leaders had both comprehension and staff, but were without ML and AI tools designed for their specific needs.
The opportunity was ripe for partnership, and Ghani embraced it. He launched the Data Science for Social Good Initiative in 2013, while working at the University of Chicago.
The program has been replicated at the University of Washington (2015), Stanford University (2019), Georgia Institute of Technology (2019), and Imperial College of London (2019), among others.
Following a pause resulting from the pandemic, the first class of 24 DSSG Fellows at CMU completed six projects in 2022.
Though the projects ranged from reducing the risk of homelessness in Pittsburgh to improving patient care in Pakistani emergency rooms, the approach to each included some common elements.
Among those key aspects: project teams are interdisciplinary. Teams consisted of individuals from different backgrounds, including computer science, ML, AI, statistics, math, economics, public policy, sociology, psychology, engineering, and physical sciences.
“None of these complex problems can be solved by any discipline alone,” Ghani said.
Another essential principle is that the projects are problem-driven. Operational challenges are identified through collaboration with project partners and community members. Project teams work closely with those directly involved with and affected by the problem as they strategize and implement solutions.
A third – and possibly the most important – component is using the lens of ethics to approach every issue.
“It’s less about ethics as a course or a lecture,” Ghani said. Instead, he explained, it’s about consistently considering the ethical implications of every decision. “What design choices are we making? What are the possible consequences of those choices downstream in three months or six months?”
Ghani says the DSSG program still has room to improve.
“Our projects are local, national, and international,” he explained. “We need to figure out better ways to be engaged with the communities in the areas affected by our projects.”
That said, it’s hard to argue with DSSG results. Data science students have an opportunity to hone their skills with support from mentors and experts while solving real-world problems. Communities and non-profit organizations bring complex challenges that they may not have the expertise or resources to tackle independently, and receive help from some of the brightest minds in the world.
And in the process, Ghani is creating a space for individuals to engage in work that they love and are good at, producing results that improve people’s lives. It turns out the work Ghani really wanted to do appeals to a whole new generation of data scientists – and he is forging the path to show them what’s possible.
The opportunity was ripe for partnership, and Ghani embraced it. He launched the Data Science for Social Good Initiative in 2013, while working at the University of Chicago.
The program has been replicated at the University of Washington (2015), Stanford University (2019), Georgia Institute of Technology (2019), and Imperial College of London (2019), among others.
DSSG at CMU: Multidisciplinary and Focused on Ethics
When Ghani returned to CMU – his alma mater – in 2019 to teach, he brought the DSSG initiative with him. DSSG Fellows spend 12 weeks working with non-profits and government agencies to tackle problems affecting real communities. Their innovative solutions have real and significant impact.Following a pause resulting from the pandemic, the first class of 24 DSSG Fellows at CMU completed six projects in 2022.
Though the projects ranged from reducing the risk of homelessness in Pittsburgh to improving patient care in Pakistani emergency rooms, the approach to each included some common elements.
Among those key aspects: project teams are interdisciplinary. Teams consisted of individuals from different backgrounds, including computer science, ML, AI, statistics, math, economics, public policy, sociology, psychology, engineering, and physical sciences.
“None of these complex problems can be solved by any discipline alone,” Ghani said.
Another essential principle is that the projects are problem-driven. Operational challenges are identified through collaboration with project partners and community members. Project teams work closely with those directly involved with and affected by the problem as they strategize and implement solutions.
A third – and possibly the most important – component is using the lens of ethics to approach every issue.
“It’s less about ethics as a course or a lecture,” Ghani said. Instead, he explained, it’s about consistently considering the ethical implications of every decision. “What design choices are we making? What are the possible consequences of those choices downstream in three months or six months?”
Ghani says the DSSG program still has room to improve.
“Our projects are local, national, and international,” he explained. “We need to figure out better ways to be engaged with the communities in the areas affected by our projects.”
That said, it’s hard to argue with DSSG results. Data science students have an opportunity to hone their skills with support from mentors and experts while solving real-world problems. Communities and non-profit organizations bring complex challenges that they may not have the expertise or resources to tackle independently, and receive help from some of the brightest minds in the world.
And in the process, Ghani is creating a space for individuals to engage in work that they love and are good at, producing results that improve people’s lives. It turns out the work Ghani really wanted to do appeals to a whole new generation of data scientists – and he is forging the path to show them what’s possible.